Revolutionizing Inventory Optimization with Snowflake
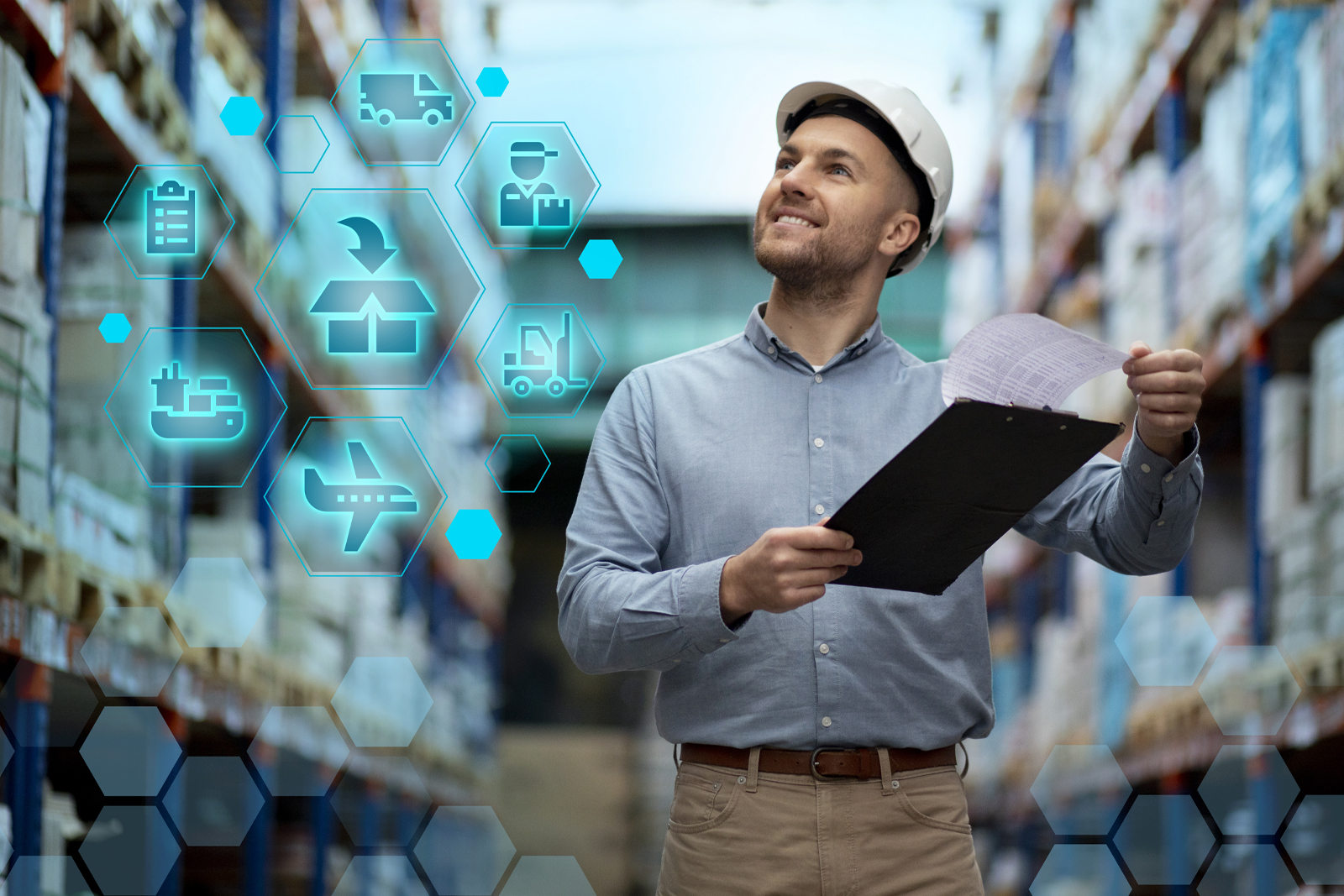
Overview
Inventory management is critical to retail success, balancing the fine line between stockouts and overstock situations. Traditional inventory systems often struggle to provide real-time insights, leading to lost sales opportunities or excess carrying costs. Snowflake’s Data Cloud empowers retailers to optimize inventory by providing real-time tracking, predictive analytics, and actionable insights to ensure optimal stock levels.
This case study explores how Snowflake helps retailers enhance inventory optimization, improve demand forecasting, and achieve operational efficiency during peak and regular seasons.
Challenges in Inventory Management
1.Lack of Real-Time Visibility
- Traditional systems often operate on batch processing, delaying insights into current inventory levels and stock movements.
- Retailers struggle to make timely decisions, leading to stockouts or overstocking.
2.Unreliable Demand Forecasting
- Historical data alone often fails to account for dynamic factors like seasonal trends, promotional impacts, and external disruptions.
- Retailers risk either underestimating or overestimating demand.
3.High Carrying Costs
- Overstock situations lead to increased warehousing expenses and potential obsolescence of unsold inventory.
- Without accurate data, inventory turnover ratios remain suboptimal.
4.Inability to Respond to Seasonal Peaks
- Retailers often face difficulties in preparing for high-demand periods, such as holiday seasons, due to inadequate predictive capabilities.
Results
- Stockouts of high-demand items were reduced by 40%, significantly improving customer satisfaction.
- Seasonal sales revenue increased by 15%, driven by better inventory availability.
- Operational costs were reduced by 20% through improved inventory allocation and reduced last-minute expedited shipping.
Detailed Requirement Capture:
+91 9597046682
How Snowflake Optimizes Inventory Management
1. Real-Time Inventory Tracking
Challenge
Delayed visibility into inventory levels leads to operational inefficiencies.
Solution
Snowflake enables retailers to integrate data from POS systems, warehouse management systems (WMS), and IoT sensors into a single, unified platform.
Outcome
Retailers can track inventory levels in real time, gaining insights into stock movements across stores and warehouses.
Increased fleet utilization rates lead to lower transportation costs and improved return on assets.
2. Predictive Analytics for Demand Forecasting
Challenge
Traditional forecasting methods struggle to account for dynamic market conditions.
Solution
Snowflake’s integration with advanced analytics and machine learning platforms enables retailers to predict demand accurately. By analyzing historical trends, real-time sales data, and external factors like weather and local events, retailers can forecast inventory needs with precision.
Outcome
Retailers ensure high-demand items are always available while minimizing excess inventory
3. Peak Season Preparation
Challenge
Insufficient inventory during high-demand seasons leads to missed revenue opportunities.
Solution
Snowflake’s scalability supports real-time analytics during peak demand periods. Predictive models powered by Snowflake anticipate customer needs, enabling timely replenishment of high-demand items.
Outcome
Retailers avoid stockouts and meet customer expectations during critical shopping seasons like Black Friday or festive holidays.
4. Optimization of Stock Levels Across Locations
Challenge
Imbalances in stock distribution across stores and warehouses result in inefficiencies.
Solution
Snowflake provides insights into regional demand patterns and stock turnover rates, allowing retailers to balance inventory across locations.
Outcome
Retailers improve inventory allocation, reduce unnecessary transfers, and lower logistics costs
5. Cost Reduction Through Efficient Inventory Management
Challenge
Overstocking increases carrying costs and risks obsolescence.
Solution
Snowflake’s analytics identify slow-moving inventory, enabling proactive markdowns or redistribution to high-demand areas.
Outcome
Retailers reduce carrying costs, improve cash flow, and enhance profitability.
Example Use Case: Ensuring Availability of High-Demand Items During Peak Seasons
- Integrated data from historical sales, customer behavior, and external factors like weather forecasts into a single platform.
- Built predictive models to identify which products would see the highest demand during peak seasons.
- Used real-time inventory tracking to monitor stock levels and automatically trigger replenishment orders.
Results and Benefits of Snowflake for Retail Inventory Optimization
1.Improved Customer Experience
- Real-time tracking and predictive analytics ensure customers find what they need when they need it, increasing loyalty.
2.Higher Revenue During Peak Seasons
- By ensuring the availability of high-demand items, retailers can capitalize on seasonal sales opportunities.
3.Reduced Inventory Costs
- Accurate forecasting minimizes overstocking and carrying costs, improving inventory turnover.
4. Enhanced Operational Efficiency
- Automated stock replenishment and optimized inventory distribution streamline supply chain operations.
5. Data-Driven Decision-Making
- Snowflake empowers retailers with actionable insights to make informed decisions, from procurement to markdowns.
Conclusion
Snowflake’s Data Cloud transforms inventory optimization for retailers, addressing long-standing challenges like stockouts, overstocking, and demand forecasting inaccuracies. By providing real-time insights and predictive analytics, Snowflake helps retailers strike the perfect balance between inventory levels and customer demand. For retailers, adopting Snowflake means not just meeting customer expectations but exceeding them, all while reducing costs and maximizing profitability. With Snowflake, inventory management evolves from a reactive process to a proactive, data-driven strategy.
More Case Studies
Raid migration using Snow Convert for an E-commerce Company
- AI Data Cloud - Snowflake/
Raid migration using Snow Convert for a Healthcare Provider
- AI Data Cloud - Snowflake/
Optimizing Inventory Management with Snowflake
- AI Data Cloud - Snowflake/
Optimizing Retail Demand Forecasting and Peak Season Readiness with Snowflake
- AI Data Cloud - Snowflake/
Transitioning SQL Server Workloads to Snowflake for Data Modernization
- AI Data Cloud - Snowflake/
Optimizing Fleet Utilization and Reducing Idle Time
- AI Data Cloud - Snowflake/